初稿
Pay Attention!!!
该文章已发表了,请勿使用该初稿投递任何期刊,它仅作为学习的参考材料。
经过对研究背景、目的、方法、结果和结论的详细阐述,我们将文章的各个部分初步整合成一篇完整的初稿。然而,需要注意的是,尽管初稿已经形成,但为了确保文章的质量和可读性,它仍需经过一系列的精修和校对过程。
结构审查:首先,我们需要对初稿的结构进行审查,确保每个部分都符合逻辑顺序,并且与研究的主题紧密相关。
内容完善:接着,对每个部分的内容进行深入的完善,包括对研究方法的详细描述、对结果的准确呈现以及对结论的有力论证。
语言润色:文章的语言需要流畅、准确,避免使用模糊不清的表达。对初稿中的语法错误、拼写错误以及不恰当的术语进行修正。
格式调整:根据目标期刊或会议的格式要求,对初稿的格式进行调整,包括引用格式、图表标注、页边距等。
同行评审:在提交前,可以让同行或导师对初稿进行评审,以获得宝贵的反馈和建议。
最终校对:根据反馈进行修改后,进行最后的校对,确保文章在提交前达到最高的学术标准。
准备提交:完成所有修订后,准备相关的提交材料,如封面信、作者信息等,以备投稿。
Title
Multiple machine learning algorithms have pinpointed SLC6A8 as a diagnostic biomarker for the late stage of Hepatocellular carcinoma.
Abstract
Introduction
Materials and methods
Data collection and download
Data Integration
Differential Expression Genes
Functional enrichment analysis
Immune cell infiltration
Potential biomarkers selection
ROC of diagnostic biomarker
Single cell transcriptome data processing and analyzing
Statistical analysis
Results
Identification of DEGs in the HCC early stage and late stage
Functional analysis of DEGs by GO and KEGG enrichment analysis
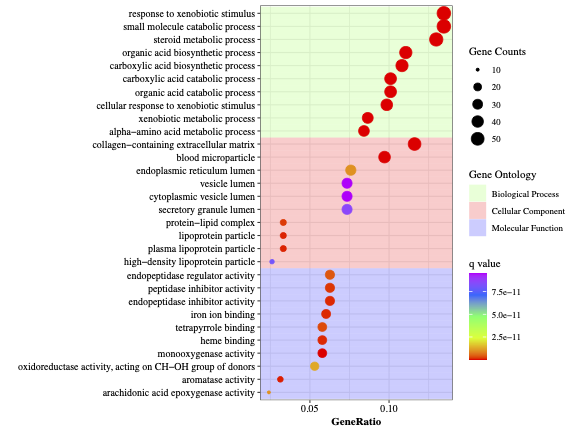
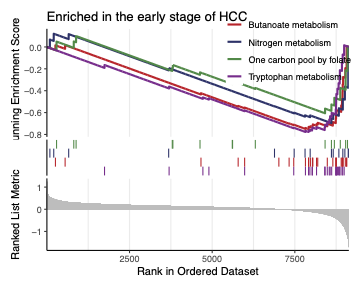
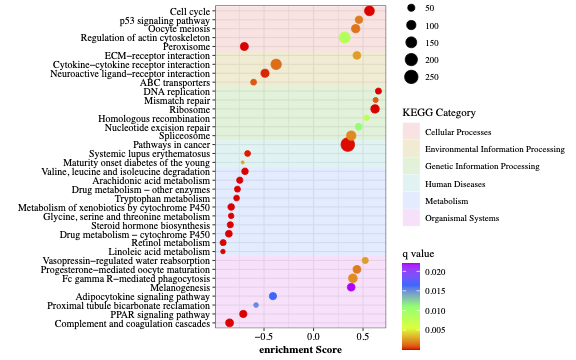
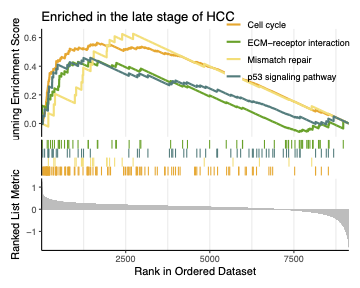
Significant changes between two stages in immune cells by ImmuneCellAI
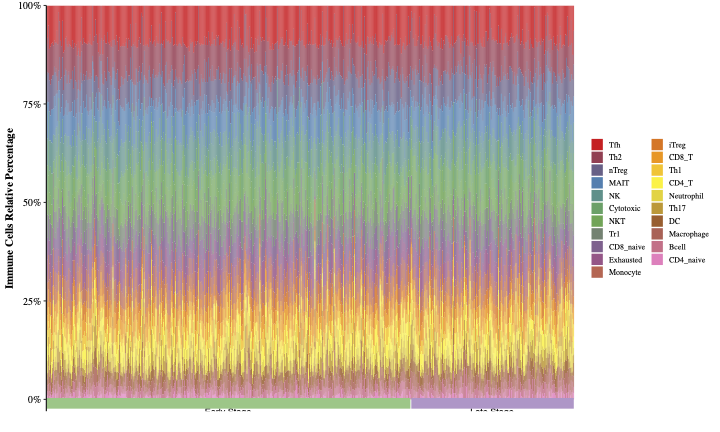
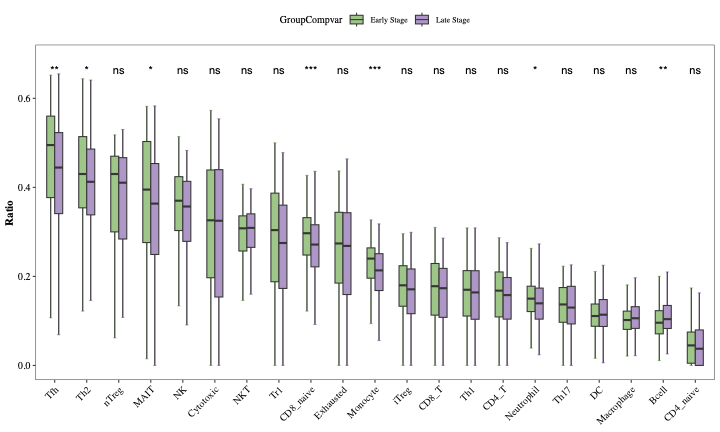
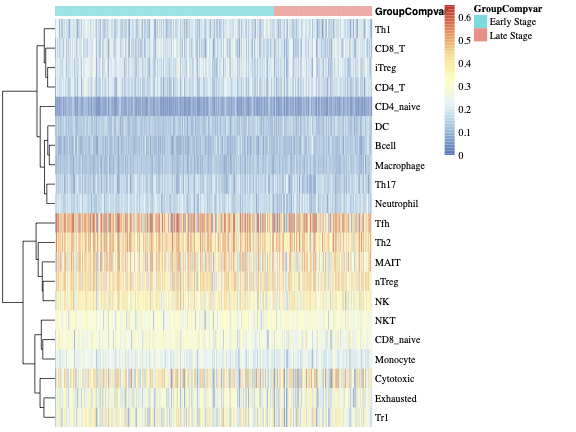
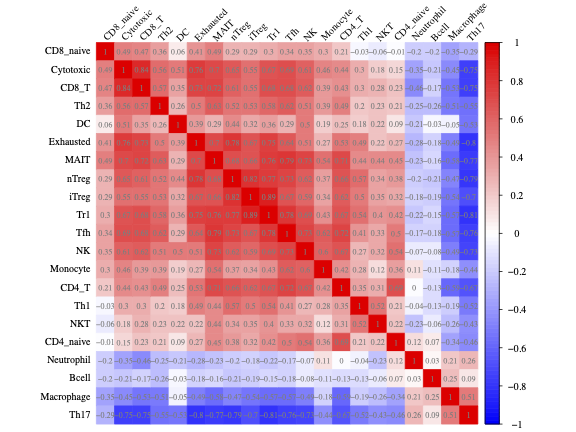
Potential gene biomarkers identified by multiple machine learning approaches
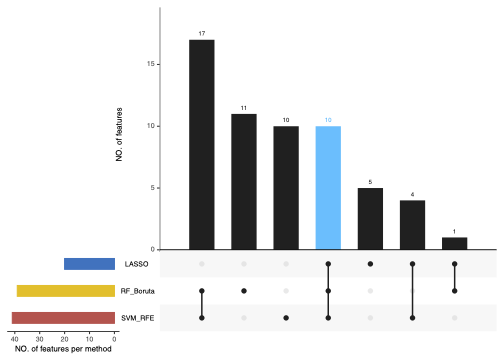
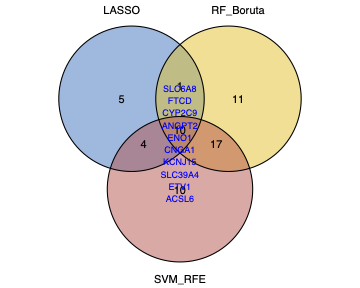
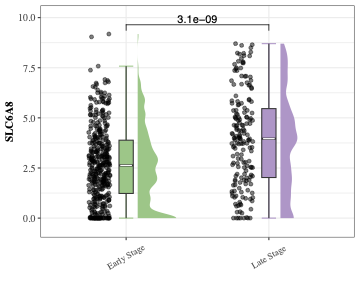
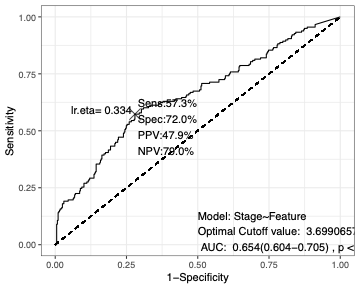
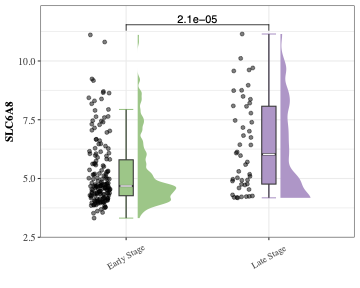
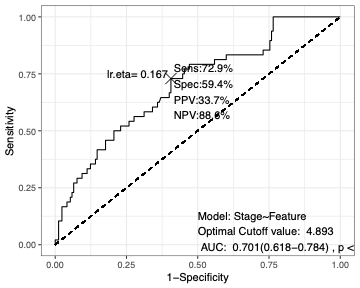
Correlation analysis between SLC6A8 and immune cells
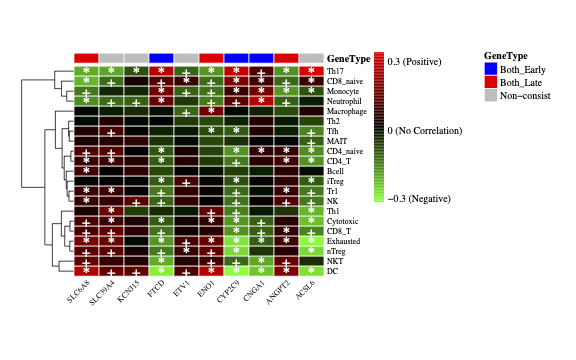
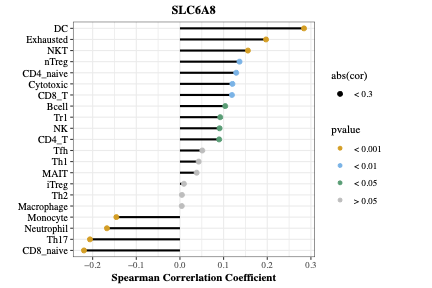
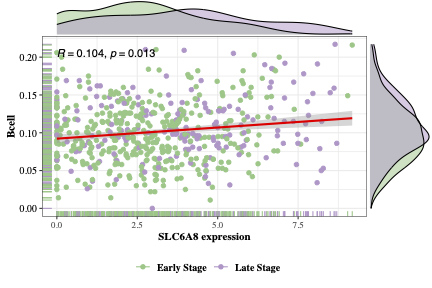
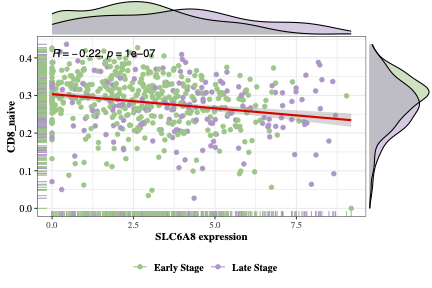
Expression level of SLC6A8 in single-cell transcriptomic data
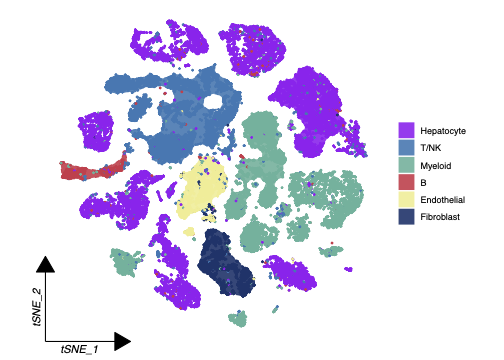
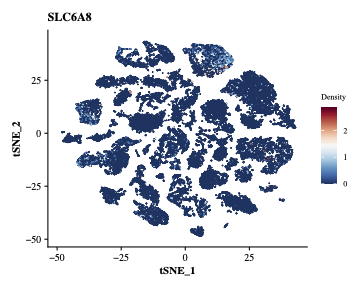
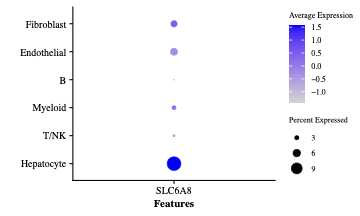
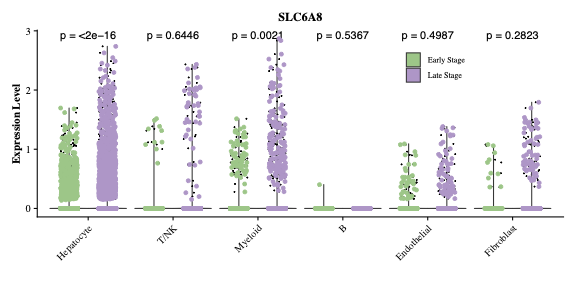